AI Colloquium
The AI Colloquium is a series of lectures dedicated to cutting-edge research in the field of machine learning and artificial intelligence, coorganized by the Lamarr Institute for Machine Learning and Artificial Intelligence (Lamarr Institute), the Research Center Trustworthy Data Science and Security (RC Trust), and the Center for Data Science & Simulation at TU Dortmund University (DoDas).
Programme
Distinguished researchers deliver captivating lectures followed by vibrant discussions. However, unlike traditional colloquia, the AI Colloquium prioritizes interactive dialogue, fostering international collaboration. Conducted primarily in English, these 90-minute sessions feature hour-long lectures and 30-minute Q&A sessions. Join every Thursday at 10 AM c.t. for a stimulating exploration of cutting-edge topics. Whether in-person at our Lecture Room on Fraunhofer Strasse 25 or via Zoom, our hybrid format ensures accessibility for all.
Day (usually) | Thursday |
Start and end time | 10 AM c.t. - 12 AM |
Duration of Presentation | 60 Minutes |
Location (usually) | Lecture Room 303 3. Floor Fraunhofer Strasse 25 Dortmund |
Upcomming Events
On early detection of hallucinations in factual question answering
- DoDas
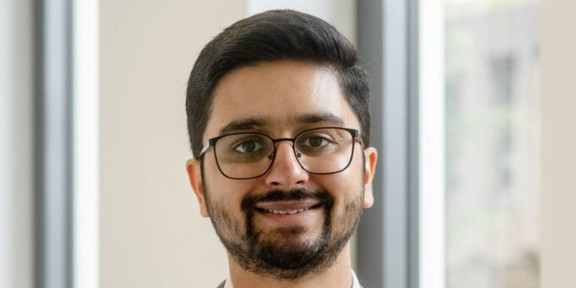
Prof. Dr. Bilal Zafar
Ruhr Universität Bochum / RC Trust
Abstract: While generative large language models (LLMs) show impressive abilities to create human like text, hallucinations remain a major impediment to their widespread adoption. In this work, we explore if the artifacts associated with the model can provide hints that a response will contain hallucinations. Specifically, we probe LLMs at 1) the inputs via integrated gradients based token attribution, 2) the outputs via the softmax probabilities, and 3) the internal state via the hidden layer and attention activations for signs of hallucinations on open ended question answering tasks. Our results show differences between hallucinations vs. non-hallucinations at all three levels, even when the first generated token is a formatting character, such as a new line. Specifically, we observer changes in entropy in input token attribution and output softmax probability for hallucinated tokens, revealing an “uncertain” behavior during model inference. This uncertain behavior also manifests itself in auxiliary classifier models trained on outputs and internal activations, which we use to create a hallucination detector. We further show that tokens preceding the hallucination can predict subsequent hallucinations before they occur.
Prof. Dr. Bilal Zafar
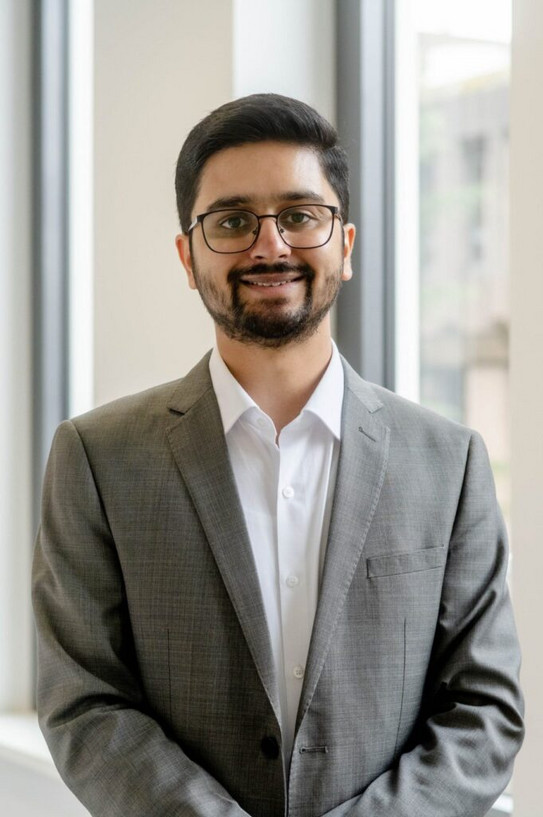
Vita: Bilal is a professor of Computer Science at Ruhr University Bochum https://informatik.rub.de/en/ and the Research Center for Trustworthy Data Science and Security http://rc-trust.ai/ . Before joining RUB, he was a Senior Scientist at Amazon Web Services where he was building products https://aws.amazon.com/sagemaker/clarify/ to support trustworthy use of AI/ML. His research interests are in the area of human-centric Artificial Intelligence (AI) and Machine Learning (ML). His work aims to address challenges that arise when AI/ML models interact with human users. For instance, he develops algorithms for making AI/ML models more fair, explainable and robust. His work has received an Otto Hahn Medal from the Max Planck Society in 2021, a nomination for CNIL-INRIA Privacy Award'18, a Best Paper Honorable Mention Award at WWW'17, a Notable Paper Award at NeurIPS'16 Symposium on ML and the Law, and a Best Paper Award at COSN'15.
Past Events
On early detection of hallucinations in factual question answering
- DoDas
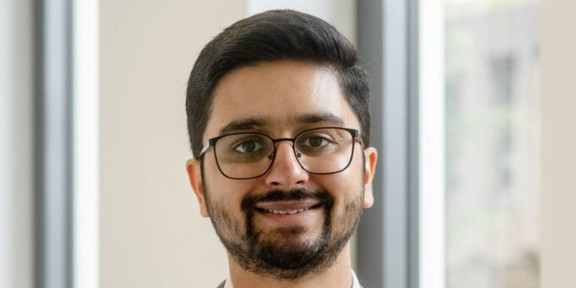
Prof. Dr. Bilal Zafar
Ruhr Universität Bochum / RC Trust
Abstract: While generative large language models (LLMs) show impressive abilities to create human like text, hallucinations remain a major impediment to their widespread adoption. In this work, we explore if the artifacts associated with the model can provide hints that a response will contain hallucinations. Specifically, we probe LLMs at 1) the inputs via integrated gradients based token attribution, 2) the outputs via the softmax probabilities, and 3) the internal state via the hidden layer and attention activations for signs of hallucinations on open ended question answering tasks. Our results show differences between hallucinations vs. non-hallucinations at all three levels, even when the first generated token is a formatting character, such as a new line. Specifically, we observer changes in entropy in input token attribution and output softmax probability for hallucinated tokens, revealing an “uncertain” behavior during model inference. This uncertain behavior also manifests itself in auxiliary classifier models trained on outputs and internal activations, which we use to create a hallucination detector. We further show that tokens preceding the hallucination can predict subsequent hallucinations before they occur.
Prof. Dr. Bilal Zafar
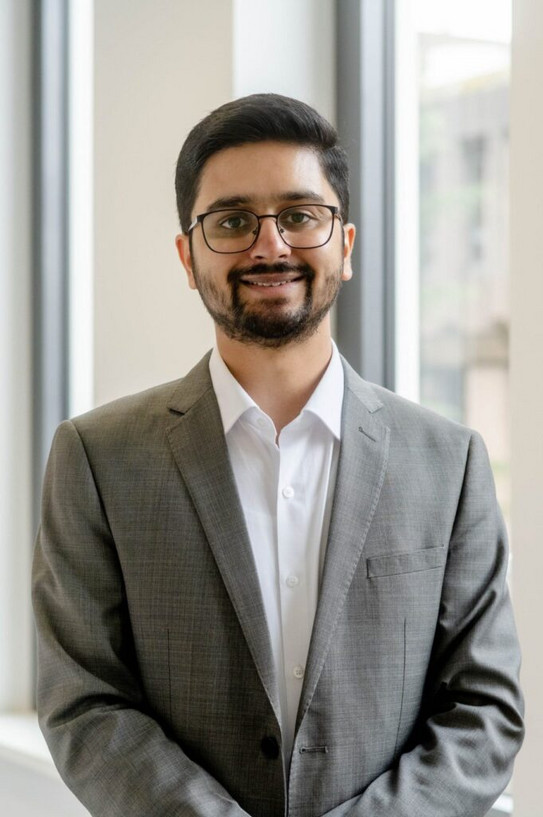
Vita: Bilal is a professor of Computer Science at Ruhr University Bochum https://informatik.rub.de/en/ and the Research Center for Trustworthy Data Science and Security http://rc-trust.ai/ . Before joining RUB, he was a Senior Scientist at Amazon Web Services where he was building products https://aws.amazon.com/sagemaker/clarify/ to support trustworthy use of AI/ML. His research interests are in the area of human-centric Artificial Intelligence (AI) and Machine Learning (ML). His work aims to address challenges that arise when AI/ML models interact with human users. For instance, he develops algorithms for making AI/ML models more fair, explainable and robust. His work has received an Otto Hahn Medal from the Max Planck Society in 2021, a nomination for CNIL-INRIA Privacy Award'18, a Best Paper Honorable Mention Award at WWW'17, a Notable Paper Award at NeurIPS'16 Symposium on ML and the Law, and a Best Paper Award at COSN'15.
On early detection of hallucinations in factual question answering
- DoDas
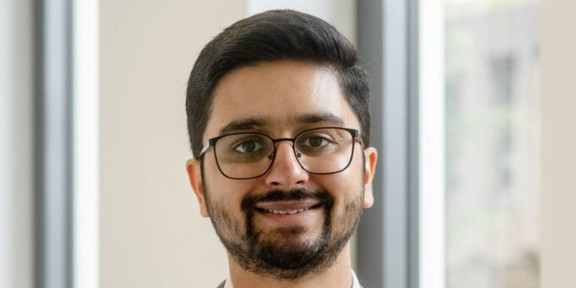
Prof. Dr. Bilal Zafar
Ruhr Universität Bochum / RC Trust
Abstract: While generative large language models (LLMs) show impressive abilities to create human like text, hallucinations remain a major impediment to their widespread adoption. In this work, we explore if the artifacts associated with the model can provide hints that a response will contain hallucinations. Specifically, we probe LLMs at 1) the inputs via integrated gradients based token attribution, 2) the outputs via the softmax probabilities, and 3) the internal state via the hidden layer and attention activations for signs of hallucinations on open ended question answering tasks. Our results show differences between hallucinations vs. non-hallucinations at all three levels, even when the first generated token is a formatting character, such as a new line. Specifically, we observer changes in entropy in input token attribution and output softmax probability for hallucinated tokens, revealing an “uncertain” behavior during model inference. This uncertain behavior also manifests itself in auxiliary classifier models trained on outputs and internal activations, which we use to create a hallucination detector. We further show that tokens preceding the hallucination can predict subsequent hallucinations before they occur.
Prof. Dr. Bilal Zafar
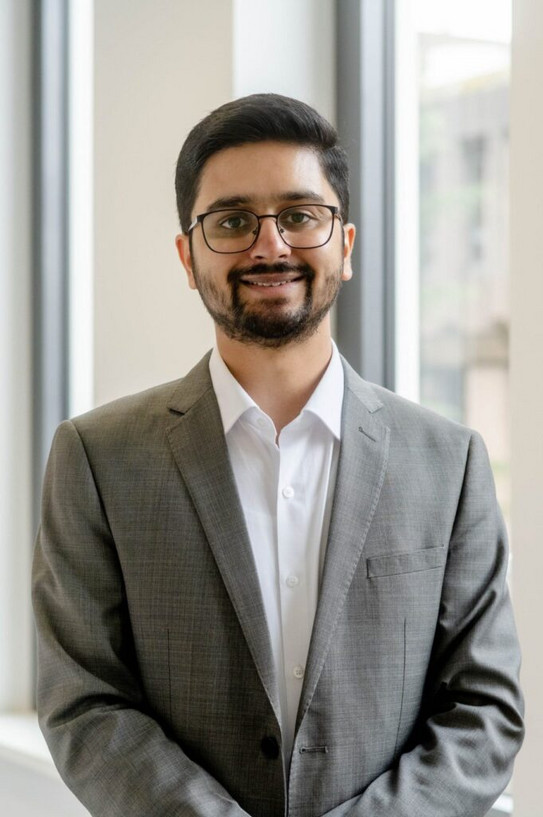
Vita: Bilal is a professor of Computer Science at Ruhr University Bochum https://informatik.rub.de/en/ and the Research Center for Trustworthy Data Science and Security http://rc-trust.ai/ . Before joining RUB, he was a Senior Scientist at Amazon Web Services where he was building products https://aws.amazon.com/sagemaker/clarify/ to support trustworthy use of AI/ML. His research interests are in the area of human-centric Artificial Intelligence (AI) and Machine Learning (ML). His work aims to address challenges that arise when AI/ML models interact with human users. For instance, he develops algorithms for making AI/ML models more fair, explainable and robust. His work has received an Otto Hahn Medal from the Max Planck Society in 2021, a nomination for CNIL-INRIA Privacy Award'18, a Best Paper Honorable Mention Award at WWW'17, a Notable Paper Award at NeurIPS'16 Symposium on ML and the Law, and a Best Paper Award at COSN'15.