AI Colloquium
The AI Colloquium is a series of lectures dedicated to cutting-edge research in the field of machine learning and artificial intelligence, coorganized by the Lamarr Institute for Machine Learning and Artificial Intelligence (Lamarr Institute), the Research Center Trustworthy Data Science and Security (RC Trust), and the Center for Data Science & Simulation at TU Dortmund University (DoDas).
Programme
Distinguished researchers deliver captivating lectures followed by vibrant discussions. However, unlike traditional colloquia, the AI Colloquium prioritizes interactive dialogue, fostering international collaboration. Conducted primarily in English, these 90-minute sessions feature hour-long lectures and 30-minute Q&A sessions. Join every Thursday at 10 AM c.t. for a stimulating exploration of cutting-edge topics. Whether in-person at our Lecture Room on Fraunhofer Strasse 25 or via Zoom, our hybrid format ensures accessibility for all.
Day (usually) | Thursday |
Start and end time | 10 AM c.t. - 12 AM |
Duration of Presentation | 60 Minutes |
Location (usually) | Lecture Room 303 3. Floor Fraunhofer Strasse 25 Dortmund |
Upcomming Events
Deterministic Clustering in High Dimensional Spaces: Sketches and Approximation
- DoDas
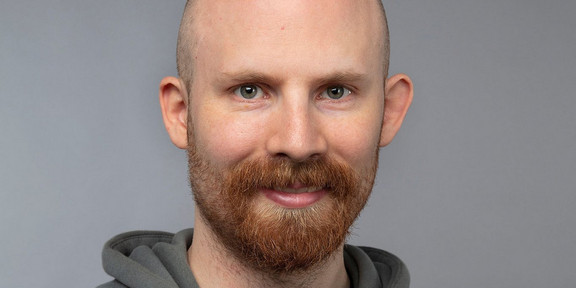
Abstract: In all state-of-the-art sketching and coreset techniques for clustering, as well as in the best known fixed-parameter tractable approximation algorithms, randomness plays a key role. For the classic k-median and k-means problems, there are no known deterministic dimensionality reduction procedure or coreset construction that avoid an exponential dependency on the input dimension d, the precision parameter ε or k. Furthermore, there is no coreset construction that succeeds with probability 1−1/n and whose size does not depend on the number of input points, n. This has led researchers in the area to ask what is the power of randomness for clustering sketches. Similarly, the best approximation ratio achievable deterministically without a complexity exponential in the dimension are Ω(1) for both k-median and k-means, even when allowing a complexity FPT in the number of clusters k. This stands in sharp contrast with the (1+ε)-approximation achievable in that case, when allowing randomization.
In this talk, we discuss deterministic sketch constructions for clustering, whose size bounds are close to the best-known randomized ones. We also show a deterministic algorithm for computing a (1+ε)-approximation to k-median and k-means in high dimensional Euclidean spaces in time 2^(k^2/ε^O(1)) poly(nd), close to the best randomized complexity. Furthermore, our new insights on sketches also yield a randomized coreset construction that uses uniform sampling, and improves over recent results by a factor k.
Prof. Dr. Chris Schwiegelshohn
Short Bio: Chris is a home grown researcher, having completed his PhD under the supervision of Christian Sohler at TU Dortmund. Subsequently, he joined Sapienza, University of Rome, first as a Postdoc hosted by Stefano Leonardi and then as a faculty member. In 2020, he joined Aarhus University where he is now associate professor in the Algorithms, Data Structures and Foundations of Machine Learning group. Chris' research focusses on algorithm design in general, with an emphasis on sketching, streaming and learning algorithms, as well as approximation and online algorithms.
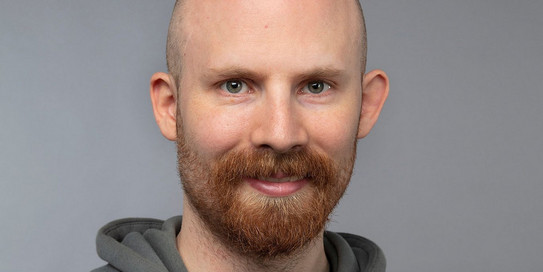
Past Events
Deterministic Clustering in High Dimensional Spaces: Sketches and Approximation
- DoDas
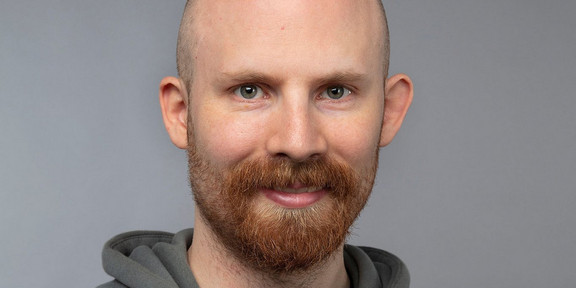
Abstract: In all state-of-the-art sketching and coreset techniques for clustering, as well as in the best known fixed-parameter tractable approximation algorithms, randomness plays a key role. For the classic k-median and k-means problems, there are no known deterministic dimensionality reduction procedure or coreset construction that avoid an exponential dependency on the input dimension d, the precision parameter ε or k. Furthermore, there is no coreset construction that succeeds with probability 1−1/n and whose size does not depend on the number of input points, n. This has led researchers in the area to ask what is the power of randomness for clustering sketches. Similarly, the best approximation ratio achievable deterministically without a complexity exponential in the dimension are Ω(1) for both k-median and k-means, even when allowing a complexity FPT in the number of clusters k. This stands in sharp contrast with the (1+ε)-approximation achievable in that case, when allowing randomization.
In this talk, we discuss deterministic sketch constructions for clustering, whose size bounds are close to the best-known randomized ones. We also show a deterministic algorithm for computing a (1+ε)-approximation to k-median and k-means in high dimensional Euclidean spaces in time 2^(k^2/ε^O(1)) poly(nd), close to the best randomized complexity. Furthermore, our new insights on sketches also yield a randomized coreset construction that uses uniform sampling, and improves over recent results by a factor k.
Prof. Dr. Chris Schwiegelshohn
Short Bio: Chris is a home grown researcher, having completed his PhD under the supervision of Christian Sohler at TU Dortmund. Subsequently, he joined Sapienza, University of Rome, first as a Postdoc hosted by Stefano Leonardi and then as a faculty member. In 2020, he joined Aarhus University where he is now associate professor in the Algorithms, Data Structures and Foundations of Machine Learning group. Chris' research focusses on algorithm design in general, with an emphasis on sketching, streaming and learning algorithms, as well as approximation and online algorithms.
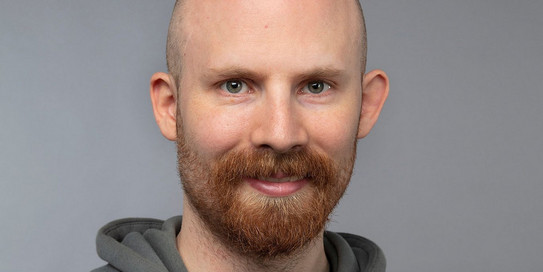
Deterministic Clustering in High Dimensional Spaces: Sketches and Approximation
- DoDas
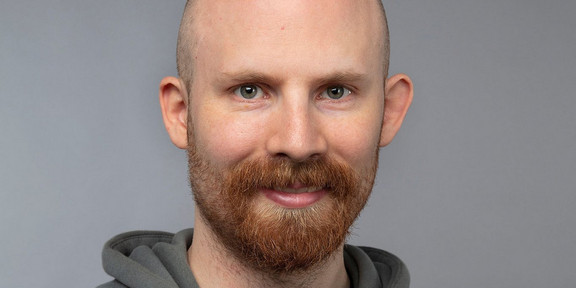
Abstract: In all state-of-the-art sketching and coreset techniques for clustering, as well as in the best known fixed-parameter tractable approximation algorithms, randomness plays a key role. For the classic k-median and k-means problems, there are no known deterministic dimensionality reduction procedure or coreset construction that avoid an exponential dependency on the input dimension d, the precision parameter ε or k. Furthermore, there is no coreset construction that succeeds with probability 1−1/n and whose size does not depend on the number of input points, n. This has led researchers in the area to ask what is the power of randomness for clustering sketches. Similarly, the best approximation ratio achievable deterministically without a complexity exponential in the dimension are Ω(1) for both k-median and k-means, even when allowing a complexity FPT in the number of clusters k. This stands in sharp contrast with the (1+ε)-approximation achievable in that case, when allowing randomization.
In this talk, we discuss deterministic sketch constructions for clustering, whose size bounds are close to the best-known randomized ones. We also show a deterministic algorithm for computing a (1+ε)-approximation to k-median and k-means in high dimensional Euclidean spaces in time 2^(k^2/ε^O(1)) poly(nd), close to the best randomized complexity. Furthermore, our new insights on sketches also yield a randomized coreset construction that uses uniform sampling, and improves over recent results by a factor k.
Prof. Dr. Chris Schwiegelshohn
Short Bio: Chris is a home grown researcher, having completed his PhD under the supervision of Christian Sohler at TU Dortmund. Subsequently, he joined Sapienza, University of Rome, first as a Postdoc hosted by Stefano Leonardi and then as a faculty member. In 2020, he joined Aarhus University where he is now associate professor in the Algorithms, Data Structures and Foundations of Machine Learning group. Chris' research focusses on algorithm design in general, with an emphasis on sketching, streaming and learning algorithms, as well as approximation and online algorithms.
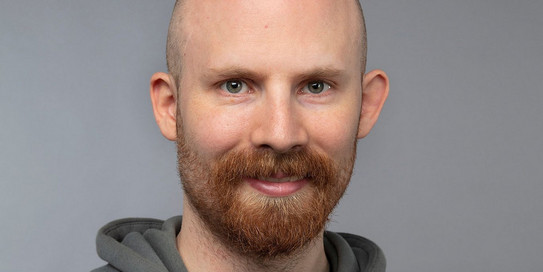