Machine Learning in Particle Physics
- Lamarr
- Physics
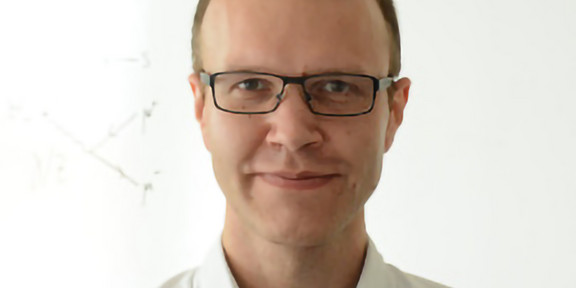
Dr. Christoph Langenbruch
Heisenberg fellow at Heidelberg University
Abstract - The enormous amount of data taken at the Large Hadron Collider offers a unique opportunity to study the fundamental building blocks of nature with unprecedented precision. At the same time, the analysis of the ever-increasing volume of data represents an enormous challenge. To address this challenge, and to optimally exploit the data, modern machine-learning methods are essential. Since any physical measurement has to be provided with a precise confidence interval, these machine-learning methods have to be accurate and robust, besides being resource-efficient. Monte Carlo simulations are an important validation tool to ensure the required performance characteristics.
In this talk, I will give an overview of machine learning techniques in particle physics with particular focus on application in the LHCb experiment, one of the four large experiments located at the LHC. The LHCb experiment recently moved its first event filter stage, the so-called trigger, to a flexible full software system, using a heterogeneous GPU+CPU architecture. This makes LHCb an ideal environment for the development and deployment of novel machine-learning methods. Besides the real-time analysis in the trigger system, machine-learning methods are furthermore used in the event reconstruction, fast simulation, particle identification, and of course offline data analysis. I will give examples of the current usage of machine-learning techniques within LHCb, discuss ongoing developments, and give a brief outlook on possible future improvements.
Dr. Christoph Langenbruch
Dr. Christoph Langenbruch is a Heisenberg fellow at Heidelberg University. He also leads an independent junior research group in the Emmy-Noether programme, which he initiated at RWTH Aachen. Previously, he held a Marie-Curie fellowship and was a research fellow at CERN. His research focuses on the analysis of rare processes in the enormous data samples taken by the LHCb experiment, located at the Large Hadron Collider at CERN. His research interests include the development and application of advanced statistical and machine-learning methods. He is furthermore working on real-time data analysis in the LHCb trigger system. In addition to his work on data analysis his research interests include the event reconstruction, where he is involved in the track reconstruction and the development of tracking detectors. He is contact author for several key analyses of the LHCb collaboration and has published on statistical topics in peer-reviewed high profile journals.
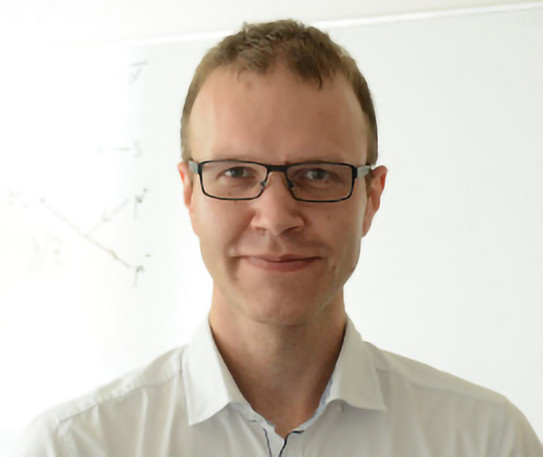