Interpretable Subgroups and Interaction Detection
- RC Trust
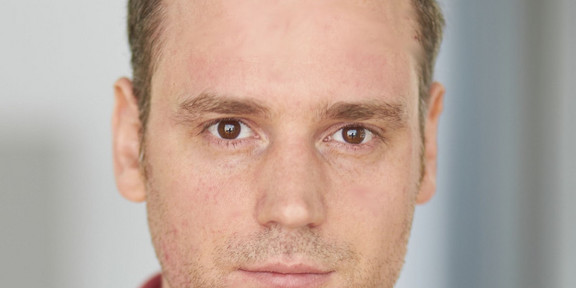
Prof. Dr. Bernd Bischl
Chair of Statistical Learning and Data Science at the Department of Statistics at the Ludwig-Maximilians-University Munich, and Co-director of the Munich Center for Machine Learning (MCML)
Abstract: Model-agnostic interpretation methods are often used in ML to produce explanations based on non-linear, non-parametric prediction models. Explanations are often represented in the form of summary statistics or visualizations, e.g., feature importance values or effects. Many interpretation methods describe the behavior of black-box models either locally for a specific observation or globally for the entire model and input space. Interpretable machine learning (IML) methods such as partial dependence plots visualize marginal feature effects but may lead to misleading interpretations when feature interactions are present. Hence, employing additional methods that can detect and measure the strength of interactions is paramount to better understand the inner workings of ML models. Furthermore, methods that produce regional explanations and lie between local and global explanations are rare and not well studied, but offer a flexible way to combine advantages between both types of explanations. Here, we will focus on subgroup approaches for IML methods, where interpretable areas in the input space are often induced by a combination of recursive partitioning and IML. Specifically, we will present regional effect plots with implicit interaction detection, a novel framework to detect interactions between a feature of interest and other features.
The framework also quantifies the strength of interactions and provides interpretable and distinct regions in which feature effects can be interpreted more reliably, as they are less confounded by interactions. In a second part, we will extend the REPID algorithm to our new framework GADGET (Generalized Additive Decomposition of Global Feature Effects), which is combinable with many existing effect estimation approaches, e.g. ALE or Shapley dependency plots.
Prof. Dr. Bernd Bischl
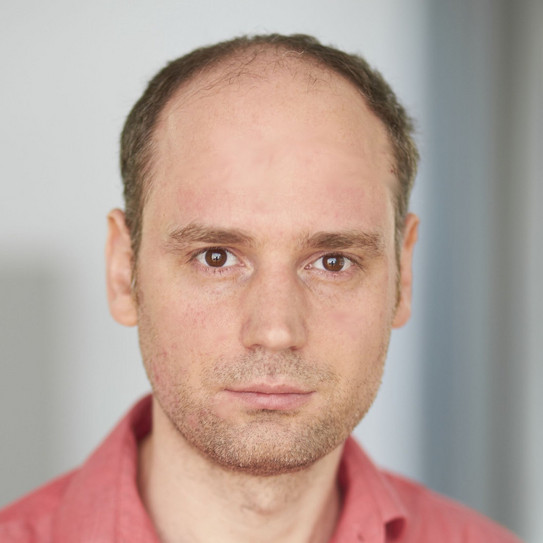
Bio: Bernd Bischl holds the chair of Statistical Learning and Data Science at the Department of Statistics at the Ludwig-Maximilians-University Munich and is a co-director of the Munich Center for Machine Learning (MCML), one of Germany’s national competence centers for ML.
He studied Computer Science, Artificial Intelligence and Data Sciences in Hamburg, Edinburgh and Dortmund and obtained his Ph.D from Dortmund Technical University in 2013 with a thesis on “Model and Algorithm Selection in Statistical Learning and Optimization”.
His research interests include AutoML, model selection, interpretable ML, as well as the development of statistical software.
He is a member of ELLIS in general, and a faculty member of ELLIS Munich, an active developer of several R-packages, leads the mlr (Machine Learning in R) engineering group and is co-founder of the science platform OpenML for open and reproducible ML.
Furthermore, he leads the Munich branch of the ADA Lovelace Center for Analytics, Data & Application, i.e. a new type of research infrastructure to support businesses in Bavaria, especially in the SME sector.